Program
Invited speakers
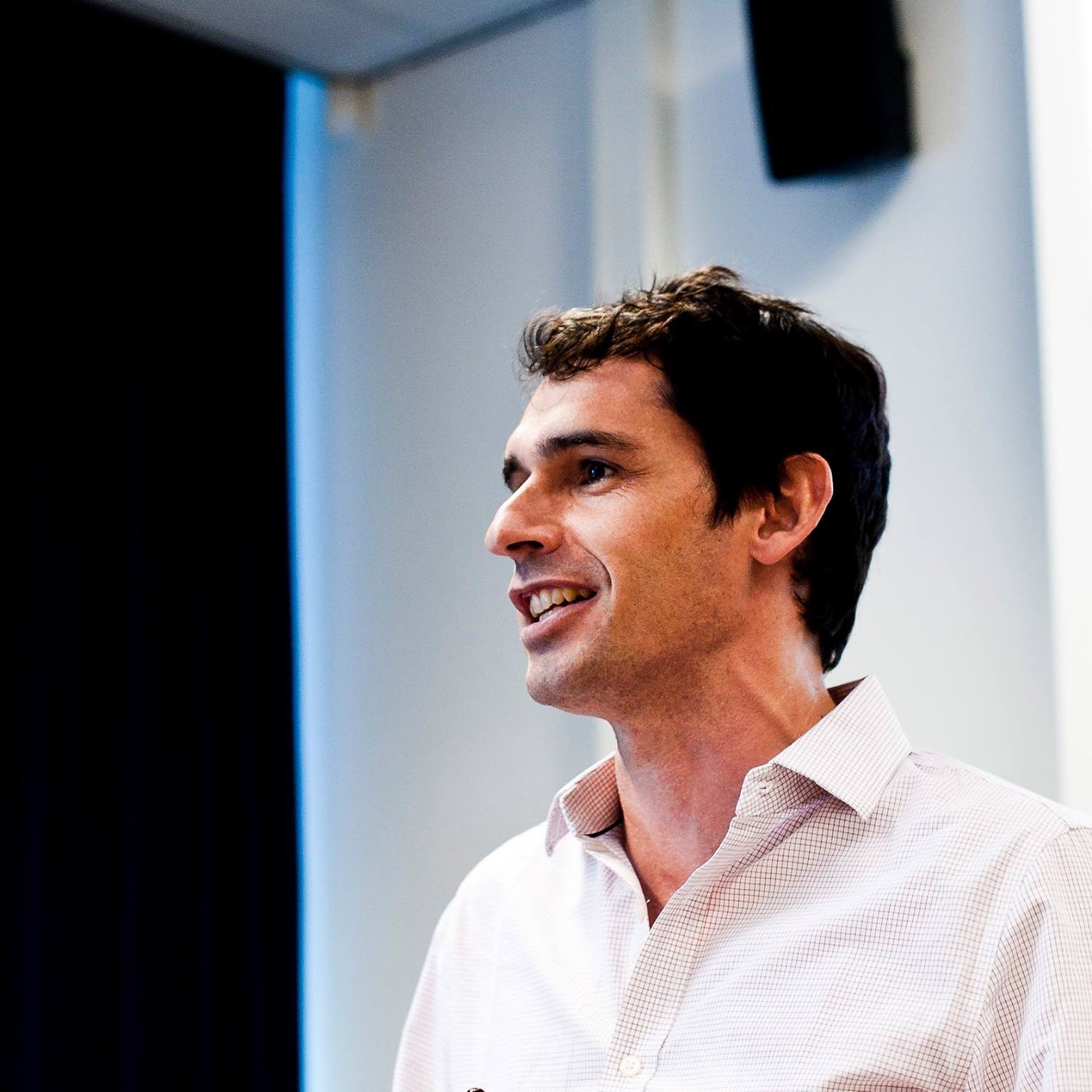
Edouard Machery
Psychological theories of concepts
Abstract: In this talk, I will review classic and more recent theories of concepts, including prototype, example, and theory theories of concepts. We will also look at the modeling of concepts in psychology by means of causal bayes network and generative, hierarchical bayesian models.
Edouard Machery is Distinguished Professor in the Department of History and Philosophy of Science at the University of Pittsburgh, the Director of the Center for Philosophy of Science at the University of Pittsburgh, a member of the Center for the Neural Basis of Cognition (University of Pittsburgh-Carnegie Mellon University), and an Adjunct Research Professor in the Institute for Social Research at the University of Michigan. He is the author of Doing without Concepts (OUP, 2009) and of Philosophy Within Its Proper Bounds (OUP, 2017).
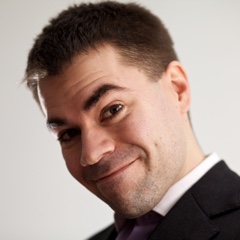
Pauli Miettinen
Boolean tensor factorizations – and beyond
Abstract: Boolean matrix factorization (BMF) has become a popular method in data mining, with applications ranging from bioinformatics to lifted inference and multi-label classification. Tensor factorizations (over the standard algebra) have gained increasing interest in data analysis community in the recent years, and they have been applied to network analysis, dynamic networks, and to simplify deep neural networks, among others. Boolean tensor factorization (BTF) – a natural combination of BMF and tensors – can be seen as a generalization of BMF, where instead of a single binary relation (i.e. a matrix), we factorize a higher-arity relation (or a collection of binary relations over the same entities). In this talk I will cover what will happen when we merge ideas from standard tensor factorizations with Boolean algebra, discussing the computational complexity, possible algorithmic ideas, and potential applications. I will also cover some hybrid approaches that merge continuous and Boolean decompositions.
Pauli Miettinen is a senior researcher and head of the area Data Mining at Databases and Information Systems department of Max-Planck-Institut für Informatik (Saarbrücken, Germany). He is also an Adjunct Professor (docent) of computer science at University of Helsinki. His main research interest is in Algorithmic Data Analysis. In particular, he is interested in applying matrix and tensor factorizations over non-standard algebras – for example, Boolean or Tropical algebras – to data mining problems. Modelling data mining problems, such as subgraph discovery, as matrix factorization problems allows to utilize existing work from these seemingly unrelated fields and gives novel insights when developing new methods. His other main branch of research is in redescription mining. In particular, the applications of redescription mining to other fields of science, such as biology, material sciences, and political science. Increasing the applicability of redescription mining or matrix and tensor methods requires advances in interactive and visual data mining; his research on interaction and visualisation naturally connects to the above topics. Pauli publishes in leading data mining journals and conferences, and three of his papers have won best paper awards. Pauli is an action editor of Data Mining and Knowledge Discovery and regularly serves in the program committees of leading data mining conferences.
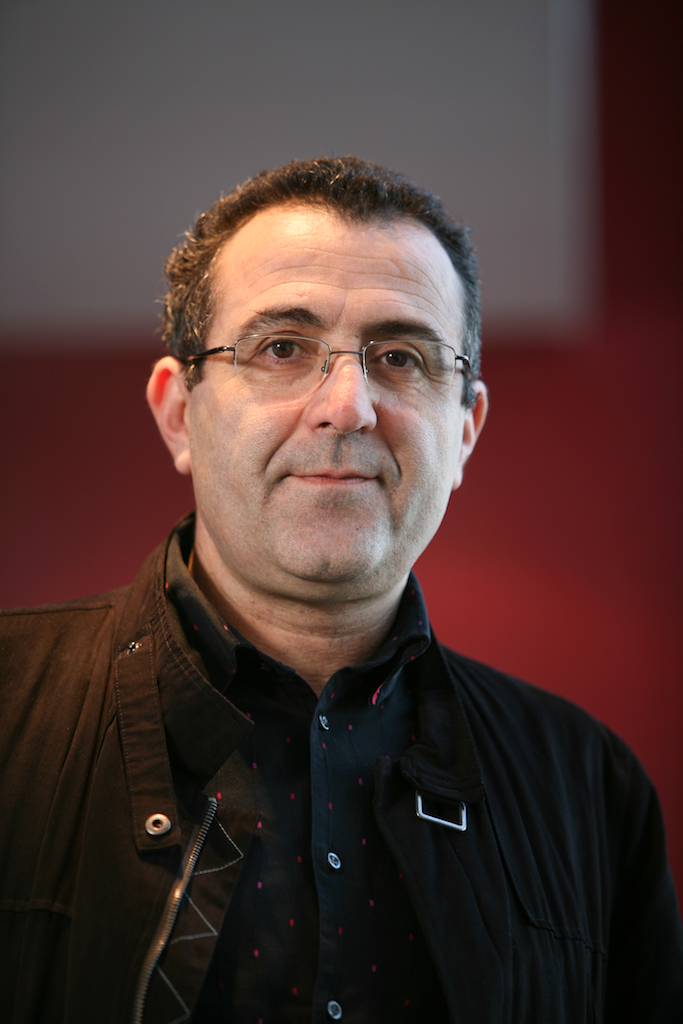
Lakhdar Sais
Towards Cross-Fertilization between Data Mining and Constraints
Abstract: In this talk, we overview our contributions to data mining and more generally to the cross-fertilization between data mining, constraint programming and propositional satisfiability. We will focus on three contributions. First, we show how propositional satisfiability (SAT) can be used to model and solve problems in data mining. As an illustration, we present a SAT-based declarative approach for itemset, association rules and sequences mining. Then, we present an original use of data mining techniques to compress Boolean formulas. Finally, we discuss how symmetries widely investigated in Constraint Programming (CP) and Propositional Satisfiability (SAT) can be extended to deal with data mining problems.
Lakhdar Sais obtained an engineering degree in computer science in 1988 from the National Institute on Computer Science ("Université de Tizi-Ouzou", Algeria), a Ph.D ("Doctorat") in 1993 from the "Université de Provence" (Marseille) and an "Habilitation à Diriger des Recherches" from the "Université d'Artois" in 2000. In 1994, he joined the "IUT de Lens" as a lecturer ("Maitre de conférences") at the beginning of the creation of the CRIL research center ("Centre de Recherche en Informatique de Lens"). Before his current position as a professor at CRIL-CNRS "Université d'Artois", he spent one year as a professor at IRIT Université Paul Sabatier (Toulouse, France). He spent two years as a researcher at INRIA Lille and CNRS. He is the founding-member and the leader (from 2002 – 2013) of the inference and decision process group at CRIL - CNRS. He is currently the Delegate director of the CRIL laboratory. His research focuses on search and representation problems in Artificial Intelligence. He is especially interested in propositional satisfiability, quantified boolean formula, constraint programming, knowledge representation and reasoning, data mining.
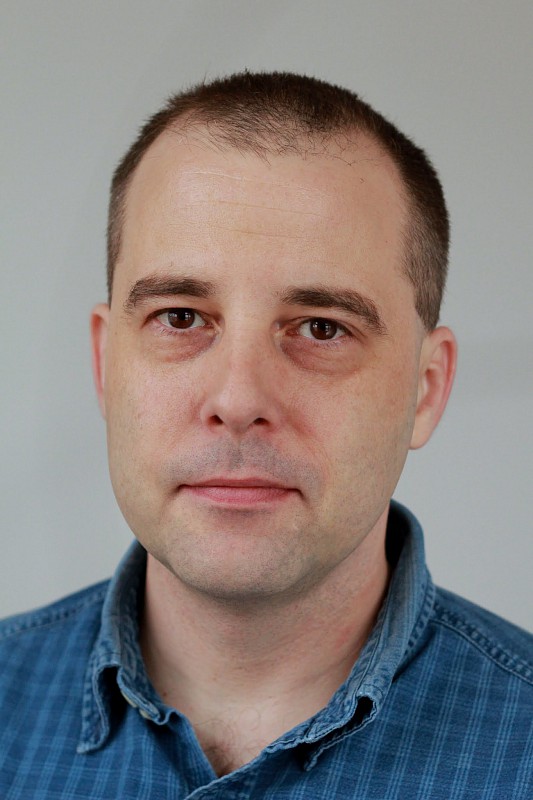
Filip Železný
Relational Machine Learning
Abstract: I will explain the main concepts of relational machine learning, or more precisely, those parts of it employing logic as the knowledge-representation formalism. The talk will not cover other relational approaches such as graph-mining. I will follow what I consider the three main stages of the field's historical development. First, I will visit the roots of relational learning lying in the area of inductive logic programming. Here, one learns logical theories from examples, formalizing the problem as search in a clause subsumption lattice. A newer stream of research called statistical relational learning extended the logical underpinnings with probabilistic inference. I will illustrate this with an example of a logical graphical probabilistic model. Most recently, relational learning has received a new impetus from the current revival of (deep) neural networks. I will exemplify some promising crossovers of the two fields, including the paradigm of Lifted Relational Neural Networks conceived in my lab.
Filip Zelezny is professor of computer science at the Czech Technical University in Prague. Previously he was post-doc at the University of Wisconsin in Madison and visiting professor at SUNY Binghamton. Currently he is head of a research lab working in the areas of machine learning, inductive logic programming and bioinformatics. He is member of the Machine Learning Journal editorial board and was the chair of the ECML PKDD 2013 conference.